High Frame-Rate Imaging Using Swarm of UAV-Borne Radars | IEEE Journals & Magazine | IEEE Xplore
Abstract: High frame-rate imaging of synthetic aperture radar (SAR), known as video SAR, has received much research interest these years. It usually operates at extremely high frequency and even THz band as a technical tradeoff between high frame rate and high resolution. As a result, video SAR system always suffers from limited functional range due to strong atmospheric attenuation of signals.
This article attempts to present a new high frame-rate collaborative imaging regime in the microwave frequency band based on a swarm of unmanned aerial vehicles (UAVs). The spatial degrees of freedom are employed to shorten the synthetic time and thus improve the frame rate. More specifically, the long synthetic aperture is split into multiple short subapertures, and each UAV-borne radar implements short subaperture imaging in a short time.
Then, the accelerated fast back-projection (AFBP) algorithm is employed to fuse multiple subimages to produce an image with high azimuth resolution. To implement the collaborative working of swarm of UAV-borne radars, a suitable orthogonal waveform is selected and a useful spatial configuration of the swarm is designed to compensate for the effect of the orthogonal waveform on imaging. Simulation results have been presented to highlight the advantages of collaborative imaging using a swarm of UAV-borne radars.
J. Ding, K. Zhang, X. Huang and Z. Xu, "High Frame-Rate Imaging Using Swarm of UAV-Borne Radars," in IEEE Transactions on Geoscience and Remote Sensing, vol. 62, pp. 1-12, 2024, Art no. 5204912, doi: 10.1109/TGRS.2024.3362630.
keywords: {Radar imaging; Radar;Imaging; Synthetic aperture radar; Apertures; Collaboration; Image resolution; Collaborative radar imaging; microwave high frame-rate imaging; radar network; synthetic aperture radar (SAR); video SAR},
URL: https://ieeexplore.ieee.org/stamp/stamp.jsp?tp=&arnumber=10428066&isnumber=10354519
Published in: IEEE Transactions on Geoscience and Remote Sensing ( Volume: 62)
SECTION I. Introduction
Radar mounted on a civil unmanned aerial vehicle (UAV) has recently entered a period of significant progress in technology innovations, as researchers continue to explore their applications, and particularly, UAV-based radar imaging has been extensively researched [1], [2]. Most recently, the use of UAV swarms is highly expected to open a gate of various new applications of radar because of high flexibility and low cost. In the past years, some successful experiments of UAV swarms have been demonstrated worldwide with an emphasis on coordinated formation flight [3], [4], [5], which inspires researchers to pay much attention to the swarm of UAV-borne radars. Unfortunately, the implementation of collaborative working of the swarm of UAV-borne radars is greatly hampered due to a few technical difficulties, such as reliable solution of system precise synchronization and dynamic communication, which differs a lot from the payloads of photoelectric sensors that have been widely mounted on UAVs. The analysis and development of the swarm of UAV-borne radars are relatively underexplored until now.
It has long been recognized that distributed collaborative radar networks with a higher degree of coordination than conventional multistatic radars could provide an entirely new paradigm to overcome fundamental limitations of today’s radars that work independently or with poor collaboration [6], [7]. However, the previous efforts in collaborative radar network are mostly restricted to ground-based radar systems with limited dynamics, technically because they strongly rely on optical fibers to ensure high-quality and high coherence of signals. This implementation becomes very difficult, if not impossible, in airborne scenarios due to the high dynamics of platforms. This article attempts to present a useful collaborative imaging regime based on a radar swarm of UAVs that can be afforded by state-of-the-art technologies.
It has been highly desired in the radar community to develop a microwave sensor with high resolution that operates as effectively as current electro-optical sensors. Video synthetic aperture radar (SAR), an area of great current activity, can provide a persistent view of the scene of interest by high frame-rate imaging [8], [9], [10]. Additionally, a moving target often leaves dynamic shadows at their true instant position in sequential images, which allows for an alternative solution for detection, location, and tracking of moving targets [11], [12]. The imaging frame rate determines what kind of target we can track. There is always a trade-off between resolution and frame rate, which pushes the operational frequency of the radar to enter the THz band [13], [14]. However, the use of such a high frequency significantly adds to atmospheric attenuation of radar signals, and therefore, the growing concern regarding video SAR is the limited functional range, which in many cases is not acceptable. It is highly expected that video SAR systems can work in classical radar bands, for example, K-band.
When video SAR operates at a relatively low frequency, there are still two approaches to achieving a high frame rate. The first is to increase the speed of the radar platform [15]. As the speed of the platform increases, the coherent processing time to obtain the desired azimuth resolution decreases, and thus, the frame rate increases. As a side effect, the azimuth Doppler bandwidth expands, which often results in the Doppler ambiguity of the SAR system. Moreover, the platform speed is not adjustable in many applications. The second approach is to improve the aperture overlapping ratio in image formation, which has been often used for obtaining a high frame rate. However, the negative result is no-return area in the SAR scene may quickly be washed out since the synthetic time of each frame is not reduced in aperture overlapping processing, which adds to the difficulty of the detection of moving target shadow.
Distributed airborne radar utilizes multiplatform to create larger synthetic aperture and can obtain high imaging resolution [16]. In other words, high-resolution images are available in a shorter synthetic duration by fusing multiview information from distributed radars. Actually, the swarm of UAV-borne radars has much higher flexibility in configuration designation than the common airborne counterparts [21], which gives rise to high frame-rate imaging without relying on aperture overlapping in classical radar bands.
This article attempts to demonstrate a collaborative radar imaging approach using the swarm of UAV-borne radars, which is an alternative for high frame-rate imaging in classical radar frequency bands rather than video SAR designed in THz frequency. The proposed approach has been verified by using simulations. The article is organized as follows. Section II briefly describes video SAR and the accelerated fast back-projection (AFBP) algorithm. Section III presents the developed framework for high frame-rate imaging based on the swarm of UAV-borne radars. The experimental examination are detailed in Section IV, and Section V concludes this article.
SECTION II. Preliminaries
A. Video SAR
Video SAR is a classical high frame-rate imaging system, which can generate sequential SAR images at a frame rate similar to conventional video formats, and the frame rate can be given as

In
conventional SAR imagery, moving targets are detected through their
Doppler energy. However, the Doppler may be shifted or smeared. In
contrast, moving target shadows precisely present their locations and
motion [23]. Consequently, we can find moving targets by detecting their dynamic shadows in video SAR image sequences [11], [12].
The performance of moving target detection by shadow is associated with
the difference between the shadow and its surrounding background. The
shadow-to-background ratio (SBHR) statistically describes the intensity
contrast between a moving target shadow and its surrounding background [12]. The smaller the SHBR, the darker the shadow, and the easier to detect. For a moving target with the length of

B. Accelerated Fast Back-Projection Algorithm
The AFBP algorithm [27]
has been developed for spotlight SAR imaging. Thanks to the unified
pseudo-polar coordinate system used in subimage formation, the AFBP can
efficiently implement image fusion via fast Fourier transform (FFT) and
circular shift, which avoids 2-D image-domain interpolation in the
classical fast back-projection (FBP) algorithm [26]. The subaperture division and the imaging coordinate system are illustrated in Fig. 1, where the platform moves along a straight flight path, generating a synthetic aperture of length
The wavenumber spectrum of the


Equations (3) and (4) reveal the linear relation between angular wavenumber
The AFBP procedure can be outlined as follows: 1) forming multiple subimages with coarse angular resolution in the unified pseudo-polar coordinate system; 2) transforming the subimages into the wavenumber domain by FFT; 3) performing spectrum center correction and spectrum connection; and 4) transforming the complete wavenumber spectrum back to image domain to obtain a full-resolution SAR image.
SECTION III. Collaborative High Frame-Rate Imaging
For the traditional SAR system with high azimuth resolution, increasing the center frequency to the W-band [17] or even THz band [13] can significantly reduce the synthetic aperture time, thereby improving frame rate and making moving target shadows easier observation. However, the signal with extremely high frequency suffers from atmospheric attenuation while the radar platform speed is limited by some physical factors. In contrast, the swarm of UAV-borne radars is completely dispersed in space, which makes it possible to shorten the synthetic time. The swarm of UAV-borne radars can be configured flexibly, which enables the multiview observation of the region of interest. Then, by fusing multiview information of multiple UAV-borne radars, high azimuth resolution can be achieved.
When each UAV-borne radar works in a self-transmitting and self-receiving mode, the collaborative imaging system based on a radar swarm of UAVs can be regarded as the collection of multiple independent UAV-borne SARs. In this case, as illustrated in Fig. 3, the long synthetic aperture to obtain high azimuth resolution can be split into multiple short subapertures, and each UAV-borne SAR implements short subaperture imaging to generate a low-resolution image. Finally, multiple low-resolution images are fused to generate an image with the desired high azimuth resolution. As a result, the synthetic aperture time is proportional to the inverse of the number of UAVs. From (9), the frame rate of the imaging system based on the swarm of UAV-borne radars can be expressed as

As opposed to video SAR, collaborative imaging based on the swarm of UAVs can reduce the synthetic aperture time

We compare the SBHR at the shadow center in SAR images obtained by the two imaging modes. As shown in Table I, compared to video SAR, collaborative imaging can reduce the SBHR when the moving target speed is greater than
SHBR curves of moving target shadow varying with target velocity and number of UAVs, where the red dotted line represents the detection requirement of SHBR (−1.5 dB).
A. Operating Mode and Waveform Design
The
basis of collaborative imaging based on the swarm of UAV-borne radars
is to transmit multiple orthogonal waveforms simultaneously, and each
waveform is associated with each UAV-borne radar. Since the
frequency-modulated continuous waveform (FMCW) is always used in
UAV-borne radars for the sake of miniaturization and lightweight, the
waveform suitable for the FMCW radar should be selected from various
orthogonal waveforms [18], [19], [20].
In FMCW radar systems, dechirping processing is employed to alleviate
sampling requirements, where a beat signal whose bandwidth is narrower
than the transmission bandwidth is generated from the backscattered
signal. Making full use of the advantage of the dechirping processing,
an orthogonal waveform named beat frequency division (BFD) is proposed [20].
Although the proposed waveform is not orthogonal during transmission,
high orthogonality can be obtained after the dechirping processing. More
specifically, when adopting the BFD waveform, as shown in Fig. 5, each UAV-borne radar transmits signals at the same chirp rate

Due to the spatial distribution of multiple UAV platforms, time-frequency synchronization errors and phase synchronization errors are inevitable in the swarm of UAV-borne radars. To reduce the effect of synchronization errors, it is crucial to choose the operating mode for the swarm of UAV-borne radars. Common distributed radar systems either operate in a one-transmitter-multiple-receiver mode or in a multiple-transmitter-multiple-receiver mode, where the transmitting and receiving oscillators are generally separated. Consequently, both the two modes require the precise calibration of synchronization errors caused by different radar local oscillators among distributed UAVs, which is extremely difficult. To alleviate the effect of synchronization errors, the swarm of UAV-borne radars in this article operates in a self-transmitting and self-receiving mode, where the transmitting and receiving ends share the same reference signal source. Consequently, the frequency and phase synchronization errors can be canceled as in a monostatic SAR, enabling coherent processing across multiple platforms. In addition, time-frequency synchronization errors have an effect on the orthogonality when the BFD waveform is adopted for collaborative imaging. More details are described by the following mathematical formulas.
When the BFD waveform is adopted, the signal transmitted from the

Since
the swarm of UAV-borne radars is spatially distributed, there are
time-frequency synchronization errors and phase synchronization errors
between different UAV-borne radars, and thus the signal transmitted from
the

The reflected signal received by the

In
the dechirping processing, the reference signal is a replica of the
transmitted waveform delayed by the time to the center of the scene, and
the reference signal of the

After dechirping, the beat signal of the

Inspecting the second exponential term in (12),
we can find that time synchronization errors and frequency
synchronization errors have an effect on the signal separation. In this
case, to ensure the interferences from other UAV-borne radars can be
easily filtered, the frequency offset

To remove the last exponential term in (12)
that represents the synchronization errors, a low pass filter is
employed to filter out the interferences from the other UAV-borne radar,
and the signal from the

After compensating for the residual video phase (RVP) [24], the filtered signal can be expressed as

For simplicity, the filtered signal can be rewritten as

Further, after compensating the reference range

B. Imaging Algorithm and Configuration Design
A simple spatial configuration of the swarm of UAVs for the collaborative imaging is shown in Fig. 6, where

However,
the center wavenumber of the echo data from different UAV-borne radars
is different due to the frequency offset. As a result, the
frequency-domain imaging algorithms, such as chirp-scaling algorithm,
polar format algorithm, cannot be applied directly to do the
collaborative imaging. In addition, since the swarm of UAV-borne radars
is spatially distributed, the multichannel reconstruction algorithm [25]
is not suitable for the collaborative imaging. By contrast, the
back-projection (BP) algorithm is capable of fusing the data from
To improve processing efficiency of the BP algorithm, the FBP algorithm [26] and the fast factorized BP (FFBP) algorithm [28] split the synthetic aperture to multiple short subapertures, and then fuse low-resolution images generated from subapertures by using 2-D interpolation. However, the interpolation inevitably brings errors and also limits the efficiency. In the Cartesian factorized BP (CFBP) algorithm [29], [30], two spectrum compressing filters are used to unfold the spectrums of low-resolution images generated from subapertures, and thus low-resolution images can be fused without interpolation to produce a high-resolution image. Unlike the CFBP projects data onto a Cartesian coordinate system, the AFBP reconstructs the image in a unified pseudo-polar coordinate system and efficiently achieves image fusion by wavenumber spectrum connection. Moreover, due to the inherent processing architecture, the AFBP can be implemented on distributed processors for further efficiency, which fits well with the spatial distribution nature of the swarm.
When the AFBP is applied to the collaborative imaging, the echo data of


The Fourier transform of

According to the principle of stationary phase, we have



Obviously,
due to the frequency offset, the 2-D wavenumber spectra of the coarse
images from different UAVs are not aligned along the radial wavenumber



The flowchart of the collaborative imaging based on the AFBP is illustrated in Fig. 7. First, in the unified ground pseudo-polar coordinate system,
SECTION IV. Simulation Results
Simulation experiments have been used to assess the performance of the proposed collaborative high frame-rate imaging approach.
We compare the collaborative high frame-rate imaging system with the video SAR system in terms of imaging performance and the synthetic aperture time. The collaborative imaging system consists of six UAV-borne radars, and the beat frequency is 40 MHz. The parameters of the first UAV-borne radar are given in Table II. The heights of other UAVs can be calculated according to (27), and the imaging geometry is shown in Fig. 8. Nine stationary point targets with the same radar cross section are present, and their positions are listed in Table III. The parameters of a traditional video SAR system are the same as those of the first UAV-borne radar.
Fig. 9(a) and (b) shows the imaging result of the traditional video SAR system with the frame rate of 2.6 and 0.43 Hz, respectively. For the video SAR system operating in a common microwave band, high frame rate and high resolution are a contradiction. Fig. 9(c) is the result of the collaborative imaging system with a frame rate of 2.6 Hz. Obviously, compared to the traditional video SAR system, the collaborative imaging system achieves a higher frame rate at the same azimuth resolution, which indicates that the collaborative imaging system enables both high frame rate and high-resolution imaging in the common microwave band rather than the THz band.
To verify the effectiveness of the adopted BFD waveform, the collaborative imaging result from the echo data without BFD demodulation is given in Fig. 10. When the BFD waveform is not adopted, the signals from six UAV-borne radars interfere with each other and thus the collaborative imaging result is not acceptable.
Additionally,
we compare and analyze the imaging performance of the collaborative
imaging system under two spatial configurations. When six UAV-borne
radars follow the same circular flight path, the imaging result in the
pseudo-polar coordinate system is given in Fig. 11(a), and the corresponding wavenumber spectrum is shown in Fig. 11(c). Meanwhile, Fig. 11(b)
displays the corresponding interpolation result in the Cartesian
coordinate system for easier observation of resolution. It can be seen
that the frequency offset leads to the misalignment of the wavenumber
spectrum along the radial wavenumber
(d) and (e) Imaging results in pseudo-polar and Cartesian coordinate systems, respectively, when the collaborative imaging system works under the designed spatial configuration.
(c) and (f) Corresponding wavenumber spectra of (a) and (d) under the two spatial configurations.
However,
it is very challenging in practice to control multiple UAVs to fly at
ideal heights precisely, and thus height deviations are inevitable. We
conduct a simulation to investigate the effect of height deviations on
imaging performance. In the simulation, the actual heights of six UAVs
are listed in Table IV, where the height deviations obey the Gaussian distribution with 1 variance and the mean of
The effect of the beat frequency offset is also a concern. The main simulation parameters are shown in Table II, and the beat frequency is reset to 30, 40, and 50 MHz, respectively. The imaging results of the collaborative imaging SAR system with different beat frequency offset are given in Fig. 13. The results have no noticeable change when the frequency offset increases thanks to the designed configuration.
Furthermore, for the collaborative imaging system with the imaging frame rate of 2.6 Hz, the influence of the number of UAVs on the image performance is investigated. Fig. 14 shows the imaging results when the number of UAVs is, respectively, 2, 4, and 6. It can be seen that as the increase of the number of UAVs, the resolution is improved. Consequently, by increasing the number of UAVs, the collaborative imaging system can obtain SAR images with both high frame rate and high resolution.
In addition, we investigate the influence of trajectory errors on imaging performance. Fig. 15(a) gives the imaging result under trajectory errors, where the energy is significantly defocusing along the azimuth direction. Fortunately, the AFBP algorithm establishes the Fourier transform relationship between the image domain and the wavenumber domain, and thus traditional auto-focusing algorithms can be employed to compensate for the effects of trajectory errors. By employing the phase gradient autofocus (PGA) [31] algorithm, we can obtain the focusing imaging result despite the presence of trajectory errors among multiple UAV platforms, as shown in Fig. 15(b). Therefore, the proposed approach in this article has the potential to mitigate the effect of trajectory errors.
Finally,
a collaborative imaging simulation is used to investigate the effect of
the number of UAVs on the moving target shadows. The collaborative
imaging results with the same resolution
SECTION V. Conclusion
Collaborative operation of airborne radar network has not received much research interest until recently. This article is the first, to the best of our knowledge, to discuss high-frame rate collaborative imaging in the research field related to the emerging technology of swarm of UAV-borne radars.
The collaborative imaging system consists of multiple self-transmitting and self-receiving UAV-borne radars. Each UAV-borne radar obtains short aperture data in a short time, and multiple aperture data are then fused to achieve the desired azimuth resolution by using the AFBP SAR algorithm. Moreover, the BFD waveform is introduced for collaborative operation of distributed UAV-borne radars, and we design a useful spatial configuration to suppress the effect of the BFD waveform on collaborative imaging. The simulation results have been presented to assess the performance of the proposed approach.
New Multi-Mode Radar Incorporates Video SAR | Unmanned Systems Technology
General Atomics Aeronautical Systems, Inc. (GA-ASI) has released the Eagle Eye radar, a new Multi-mode Radar (MMR) that has been installed and flown on a U.S. Army-operated Gray Eagle Extended Range (GE-ER) Unmanned Aerial Vehicle (UAV).
Eagle Eye is a high-performance radar system that delivers high-resolution, photographic-quality imagery that can be captured through clouds, rain, dust, smoke and fog at multiple times the range of previous radars. It’s a drop-in solution for GE-ER and is designed to meet the range and accuracy to Detect, Identify, Locate & Report (DILR) stationary and moving targets relevant for Multi-Domain Operations (MDO) with Enhanced Range Cannon Artillery (ERCA). Eagle Eye radar can deliver precision air-to-surface targeting accuracy and superb wide-area search capabilities in support of Long-Range Precision Fires.
Featuring Synthetic Aperture Radar (SAR), Ground/Dismount Moving Target Indicator (GMTI/DMTI), and robust Maritime Wide Area Search (MWAS) modes, Eagle Eye’s search modes provide the wide-area coverage for any integrated sensor suite, allowing for cross-cue to a narrow Field-Of-View (FOV) Electro-Optical/Infrared (EO/IR) sensor.
The Eagle Eye’s first flight on the Army GE-ER aircraft took place in December 2021, incorporating the new Video SAR capability. Video SAR enables continuous collection and processing of radar data, allowing persistent observation of targets day or night and during inclement weather or atmospheric conditions. In addition, Eagle Eye’s processing techniques enable three modes – SAR Shadow Moving Detection, SAR Stationary Vehicle Detection and Moving Vehicle Detection as part of its Moving Target Indicator – to operate simultaneously.
“The Video SAR in Eagle Eye provides all-weather tracking and revolutionizes precision targeting of both moving and stationary targets at the same time,” said GA-ASI Vice President of Army Programs Don Cattell. “This is a critical capability in an MDO environment to ensure military aviation, ground force and artillery have constant situational awareness and targeting of enemy combatants.”
GA-ASI Introduces New Eagle Eye Radar
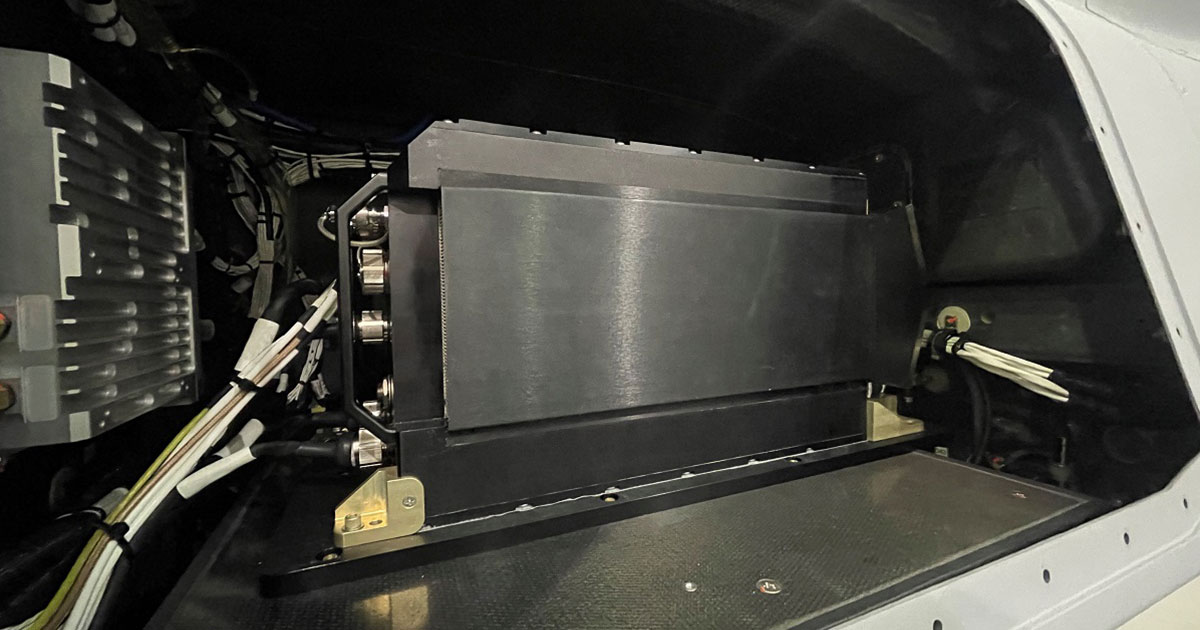
New Radar Flies on U.S. Army Gray Eagle UAS; Features New Video SAR Capability
SAN DIEGO – 05 April 2022 – General Atomics Aeronautical Systems, Inc. (GA-ASI), a leader in Multi-mode Radar technology for Unmanned Aircraft Systems, introduces the Eagle Eye radar. The new MMR is installed and has flown on a U.S. Army-operated Gray Eagle Extended Range (GE-ER) UAS. Eagle Eye joins GA-ASI’s line of radar products, which includes the Lynx® MMR.
Eagle Eye is a high-performance radar system that delivers high-resolution, photographic-quality imagery that can be captured through clouds, rain, dust, smoke and fog at multiple times the range of previous radars. It’s a “drop-in solution” for Gray Eagle ER and is designed to meet the range and accuracy to Detect, Identify, Locate & Report (DILR) stationary and moving targets relevant for Multi-Domain Operations (MDO) with Enhanced Range Cannon Artillery (ERCA). Eagle Eye radar can deliver precision air-to-surface targeting accuracy and superb wide-area search capabilities in support of Long-Range Precision Fires.
Featuring Synthetic Aperture Radar (SAR), Ground/Dismount Moving Target Indicator (GMTI/DMTI), and robust Maritime Wide Area Search (MWAS) modes, Eagle Eye’s search modes provide the wide-area coverage for any integrated sensor suite, allowing for cross-cue to a narrow Field-of-View (FOV) Electro-optical/Infrared (EO/IR) sensor.
The Eagle Eye’s first flight on the Army GE-ER aircraft took place in December, incorporating the new Video SAR capability. Video SAR enables continuous collection and processing of radar data, allowing persistent observation of targets day or night and during inclement weather or atmospheric conditions. In addition, Eagle Eye’s processing techniques enables three modes – SAR Shadow Moving Detection, SAR Stationary Vehicle Detection and Moving Vehicle Detection as part of its Moving Target Indicator – to operate simultaneously.
“The Video SAR in Eagle Eye provides all-weather tracking and revolutionizes precision targeting of both moving and stationary targets at the same time,” said GA-ASI Vice President of Army Programs Don Cattell. “This is a critical capability in an MDO environment to ensure military aviation, ground force and artillery have constant situational awareness and targeting of enemy combatants.”
About GA-ASI
General Atomics-Aeronautical Systems, Inc. (GA-ASI), an affiliate of
General Atomics, is a leading designer and manufacturer of proven,
reliable remotely piloted aircraft (RPA) systems, radars, and
electro-optic and related mission systems, including the Predator® RPA
series and the Lynx® Multi-mode Radar. With more than seven million
flight hours, GA-ASI provides long-endurance, mission-capable aircraft
with integrated sensor and data link systems required to deliver
persistent flight that enables situational awareness and rapid strike.
The company also produces a variety of ground control stations and
sensor control/image analysis software, offers pilot training and
support services, and develops meta-material antennas. For more
information, visit www.ga-asi.com
Avenger, Lynx, Predator, SeaGuardian and SkyGuardian are registered trademarks of General Atomics Aeronautical Systems, Inc.
ga-asi Apr 5, 2022
No comments:
Post a Comment